Match Point: Matching Patients at Community Sites with Clinical Trials
Helynx, Inc., in collaboration with Dr. Jae Kim
We proposed to increase clinical trial accrual among patients visiting City of Hope’s community locations. Our system parses natural language in both the trial description and the patient record, and identifies opportunities for matches which can help the general oncologist recommend a trial. The overall goal is to increase clinical trial accrual from among a population of patients who might not otherwise enroll.
After interviewing multiple oncologists at two of City of Hope’s satellite clinics, we found that the primary difficulty behind recommending patients for trials was that trials were hard to find. Oncologists at these sites are highly knowledgeable about each of their patients, and have developed effective ways of understanding the patient state at a glance. In contrast, existing clinical trials search tools oblige them to spend a great deal of time hunting through unstructured text.
We have solved this problem using our machine-based parsing of the unstructured information in the trials. The extracted information is human curated and checked for accuracy within our knowledge graph. From there, we generate a search tool from which the oncologist can find the best match in a matter of seconds.
This platform (see figure) is currently undergoing cycles of review and revision, and we hope to offer the first version to City of Hope oncologists in a month or so. Since it does not require protected health information, it can be distributed widely. Note also that is useful in contexts where relevant patient information is not in the electronic health record, such as in the first encounter with a patient. We believe this system is the most likely vehicle to increase trial accrual in the next year.
Simultaneously, we are preparing the next phase of the platform, in which the system polls the EHR and recommends patient matches in an automated way. Other attempts at automated clinical trials matching via natural-language processing systems have found varying degrees of accuracy, which can be improved by human curation within a knowledge graph. We intend to present research results at a Southern California machine learning conference this winter.
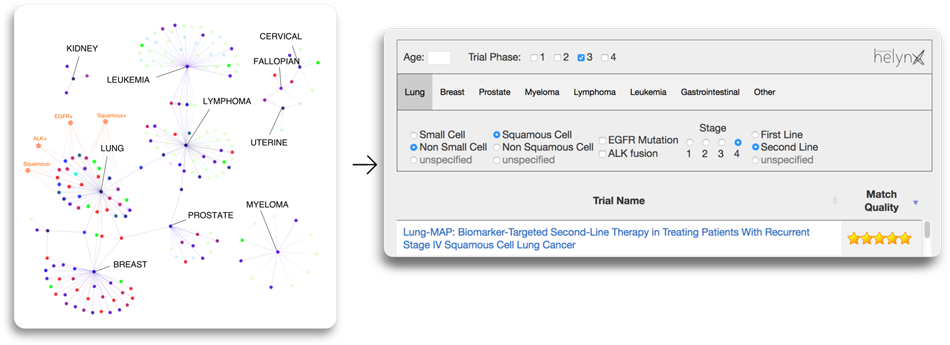
A clinical trials knowledge graph (left) deployed as a dashboard for oncologists to find the right clinical trial in seconds (right, screenshot of software in development). Our knowledge graph is derived from City of Hope’s clinical trials using machine-based techniques, and then human curated to ensure nearly perfect accuracy in parsing unstructured text.